How Machine Learning Is Shaping the Retail Fashion Industry
How Machine Learning Is Shaping the Retail Fashion Industry
How Machine Learning Is Shaping the Retail Fashion Industry
3 Abr 2023
Aptean Staff Writer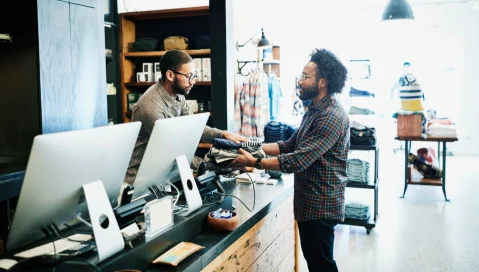
Unprecedented change is the new normal in the fashion industry. Amid the highest inflation rate in a generation, global social unrest, changing consumer behavior, rising demands for climate justice, and continued disruptions to global supply chains, retail is in a volatile state. All of these factors, plus the recent pandemic have acted as a catalyst for digital transformation, with many brands and retailers modernizing their IT ecosystems and integrating their retail systems in order to unlock increased agility and future-proof their success.
But, with digital transformation, comes an abundance of data—both a blessing and a curse for many organizations. New exponential data sources, including RFID, in-store sensors, social media and ultra-local climate records provide rich sets of information, too large to be able to humanly analyze, meaning you may miss out on the valuable insights that data holds.
Enter machine learning. This type of artificial intelligence provides the opportunity for brands and retailers to make better decisions based on reliable forecasts, to get the right inventory, in the right location, at the right price.
As a result, we are starting to witness The Great Data Schism, where brands and retailers who have adopted machine learning for demand forecasting are overperforming, while other, major well-known retailers struggle with excess inventory and markdowns, hurting their margins and profitability.
So, to help ensure your organization is not one those left behind, let’s take a more detailed look at machine learning in the fashion industry and how Aptean technology can help.
The Use of Machine Learning in Fashion and Apparel
Machine learning has been used in retail for several decades, the first widespread use in retail was in the early 2000s, when large retailers such as Amazon and Netflix began using machine learning algorithms to personalize their product recommendations for customers.
However, demand forecasting for apparel remains difficult, as fashion products have short life cycles, with trends and styles constantly changing, making it challenging to accurately predict demand for new and seasonal collections. Consumer behavior is also influenced by social media, celebrity fashion and increasingly frequent extreme weather events.
There have been several notable examples of machine learning being used in the fashion industry. For example, in 2016, the fashion brand H&M used machine learning algorithms to analyze customer preferences and design new clothing collections accordingly. Similarly, in 2018, Tommy Hilfiger partnered with the tech company IBM to create a machine learning system that could predict which items in a new collection would be most popular with customers.
Since then, machine learning has been used extensively in the retail industry for a variety of applications, including demand forecasting, inventory management, price optimization, fraud detection and customer segmentation. With the increasing availability of data and advances in machine learning algorithms, the use of machine learning in retail is only expected to grow in the coming years.
Solving the $4.3 Billion Dollar Problem
In 2018, H&M struggled with a mountain of $4.3 billion of unsold shirts, dresses and accessories. The success of the fast-fashion business model relies on anticipating fashion trends accurately and bringing them to market quickly. When a company fails to accurately predict trends and consumer preferences ahead of its competitors and/or experiences excessively long lead times, the result is a huge stockpile of unsold inventory. H&M decided to improve its ability to predict customer demand for different products across a vast global supply chain with forecasting using machine learning.
The traditional method of demand forecasting relied on historical sales data and intuition, which was often inaccurate and unreliable. With the help of machine learning, H&M aimed to develop a more sophisticated and data-driven approach to demand forecasting that could take into account a wide range of variables, including product attributes, seasonality, pricing and regional trends. By accurately forecasting demand, H&M could optimize its inventory management, reduce waste and improve the overall customer experience.
Another well-documented account of using machine learning for demand forecasting in fashion is Zara. Like many other fashion retailers, Zara faced challenges in accurately predicting customer demand for its products, particularly for new and seasonal collections. Zara now uses machine learning algorithms to analyze sales data, social media trends and customer feedback to predict what products will be popular in different regions. This helps the brand optimize inventory and reduce waste.
Stitch Fix, an online personal styling service, uses machine learning algorithms to analyze customer feedback and data on previous boxes to make personalized recommendations for future shipments. By using machine learning to predict which items would be most appealing to individual customers, Stitch Fix was able to increase customer satisfaction and retention.
Stitch Fix also uses machine learning for other applications, such as inventory management and product design. Data on customer preferences and feedback is used to not only inform decisions on which items to produce and stock but also to optimize product designs based on customer feedback.
Adidas has been using machine learning to forecast demand for its products since 2016. The brand uses a combination of historical sales data, social media trends and external factors like weather to predict demand. This has helped to reduce inventory costs and improve supply chain efficiency.
The importance of being able to share and digest data across silos for fashion retailers is best illustrated by Nike, which has acquired four data analytics companies over the last few years. Zodiac, which focuses on consumer analytics, and Invertex, the computer vision specialist were acquired in 2018, the predictive analytics company Celect in 2019, and the data integration and machine learning platform Datalogue in 2021.
A Glass Box Approach that Builds Trust
While there have been many successful applications of machine learning in retail, there remains general distrust in handing over decision making to machines due to notable failures. Machine learning algorithms are often used to predict demand for products, which can inform inventory management and supply chain decisions. However, if the algorithm is trained on faulty data, or relies too heavily on historical data, the predictions can be wildly inaccurate or heavily biased.
At Aptean, we recommend a glass box approach to demand forecasting. The glass box concept can help buyers, planners and allocators understand how the machine learning model arrived at its predictions. The glass box elements which we provide in our retail planning platform include:
A single source of truth for all your retail planning data: historical sales data, stock, intake and markdown data are stored in our retail planning data warehouse and readily available to be used to train the machine learning models.
Data affinity clusters: the data is cleaned and clustered based on affinities specific to the fashion and apparel industry, including product seasonality, Pareto analysis of sales, data sparsity and merchandise attributes.
Human intuition to adjust settings: the solution comes with recommended settings for the forecasting model. Dashboards and data visualizations allow the demand forecasters to understand the data and choice of machine learning algorithm.
Model evaluation: the performance of the model is displayed using the common forecasting accuracy metrics, including Mean Absolute Percentage Error (MAPE) which measures the average percentage difference between the actual and predicted values, and Root Mean Squared Error (RMSE), which measures the square root of the average of the squared differences between the actual and predicted values.
Model interpretation: we use methods such as feature importance and partial dependence plots to understand how the model is making its predictions and understand the interactions between variables.
Deployment and monitoring of the model in production: the model is deployed and performance is continuously monitored to ensure it remains accurate and effective.
Forecast as a Service for the Planning Ecosystem
Retailers like you rely on industry-specific solutions that can provide significant financial results by leveraging your business trends insights and historical data to strengthen the backbone—and accuracy—of your planning process.
Demand forecasting and machine learning capabilities are integrated into our retail planning software to provide a more streamlined and efficient solution. Buyers, planners and demand forecasters no longer have to import forecasts from other applications, which saves time and increases accuracy. Advanced functionalities support the delivery of the single version of truth provided by the Aptean Retail Planning data warehouse which is integrated with other data sources to increase the accuracy of our forecasts. Aptean delivers demand forecasting and machine learning as a service via the retail planning ecosystem, to wherever the teams need a forecast, within Merchandise Financial Planning, Assortment Planning, and Allocation & Replenishment.
Forecast as a Service supports a variety of use cases, which include:
Generating demand forecasts to support store replenishment.
Optimizing In-season Assortment Planning through weekly analysis of past, actual and forecast sales.
Intelligent automated re-order for core product ranges to replenish warehouses and distribution centers.
Management of never out-of-stock collections.
Informing Pre-Season and In-Season Open-to-Buy decisions.
Our planning platform provides an effective approach to demand forecasting using machine learning, helping your fashion and apparel business meet its specific challenges, leveraging the collective wisdom gleaned from thousands of retail implementations across the globe and our relentless desire to challenge conventional methods—and dispute outdated philosophies. As relentless problem solvers, we immerse ourselves fully in the challenges of retail to deliver nothing less than the absolute best.
Ready to generate highly accurate retail forecasts for your fashion and apparel brand? Contact us today to learn more about Aptean Retail Planning.
Empiece a transformar su negocio de moda y confección hoy mismo
Si está preparado para dar el siguiente paso, nos encantaría ayudarle.
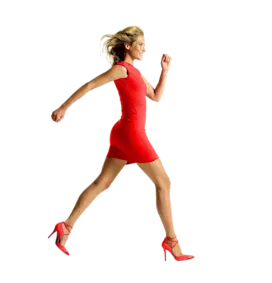